We’re excited for AI to expand the <$1T software TAM into the massive >$10T global services market. But to do that, AI needs to match more human capabilities, like answering calls, talking, negotiating, scheduling, and more. For AI to truly fulfill its TAM-expanding promise, it must be able to listen, understand, and speak more like a human.
The good news is it’s starting to happen. App builders are piecing together AI components to deliver a better conversational experience. The main components include:
- Speech-to-text: Transcription platforms like Fireflies and Granola use models from Assembly and Deepgram to capture human voice accurately and turn it into text. Â
- Text-to-text reasoning: Companies like OpenAI, Google, and Anthropic have made significant strides in conversational intelligence, and have made models capable of deep thinking.
- Text-to-speech: Players like ElevenLabs and Cartesia have reached substantial valuations, driven largely by rapid adoption of their high-quality voice synthesis tools. We’ve also seen a vanguard of open-source text-to-speech projects like ChatTTS, Dia, and OpenVoice v2 that have offered developers incredible flexibility. Google recently announced native speech generation and live audio generation, which makes it possible for a model to sound even more natural (including whispering).
While individual models are promising, there’s still friction. Most developers don’t want to cobble together multiple models. Add in components like phone number provisioning, multilingual support, translation, and more, and the prevailing voice AI stack can be a messy web of tools.
This creates two big opportunities in voice AI: true speech-to-speech models, and “Voice in a Box.”
‍
True speech-to-speech models
Voice agents that lack prosody (that is, tone, timing, inflection, and pauses) feel one-size-fits-all, limiting their ability to deliver truly personalized experiences. While today's models are increasingly accurate with words, they often miss the intent behind them. Prosody and emotion are the missing layers in voice AI. They're what turn correct AI responses into "human" ones.
Without prosody modeling, voice agents struggle to interpret context and emotional nuance. With it, they can better understand the user's emotional state and adjust their response accordingly; for example, speaking more quickly if the user sounds frustrated, or more calmly if they seem anxious.
Capturing and preserving prosody and emotion across languages remains one of the hardest unsolved challenges in voice AI. The next major breakthrough will come from large-scale, emotion- and prosody-labeled audio datasets across languages, enabling models to genuinely understand and convey tone, mood, and feeling.
We expect we’ll have voice models that natively understand and generate speech, language, and prosody in one loop. End-to-end models can preserve intonation, cadence, and timing better. Multimodal fusion lets the model interpret not only what is said, but also how and why. These models will generate speech directly with emotion, rather than stitching it on afterward. A fused model could do it all in one pass while reducing latency, which is crucial for real-time interaction.
Today, many conversational AI applications rely on modular three-step architectures that are controllable, auditable, and compliant for enterprise use. They allow for validation at each stage and are easier to debug. While multimodal fusion models will be a black box that will make it harder to isolate errors or easily verify outputs, they will also bring forth the future of more emotional and human-like experiences. Multimodal speech-to-speech models are when AI starts sounding (and acting) human.
‍
Voice in a Box
Whether we move toward a multi-model stack or an end-to-end speech-to-speech future, we believe there will be value in the “Voice in a Box” layer—the packaging and API infrastructure that makes voice AI easy to deploy.
The key reason is that building effective voice AI applications requires much more than just a model. The real complexity begins after deployment: managing phone numbers, scaling calls, handling translation, enabling custom-branded voices, voice cloning, and more. These operational needs make a streamlined, developer-friendly voice layer essential. As models continue to rapidly outpace one another in performance, this packaging layer becomes the stable, enduring part of the stack.
‍
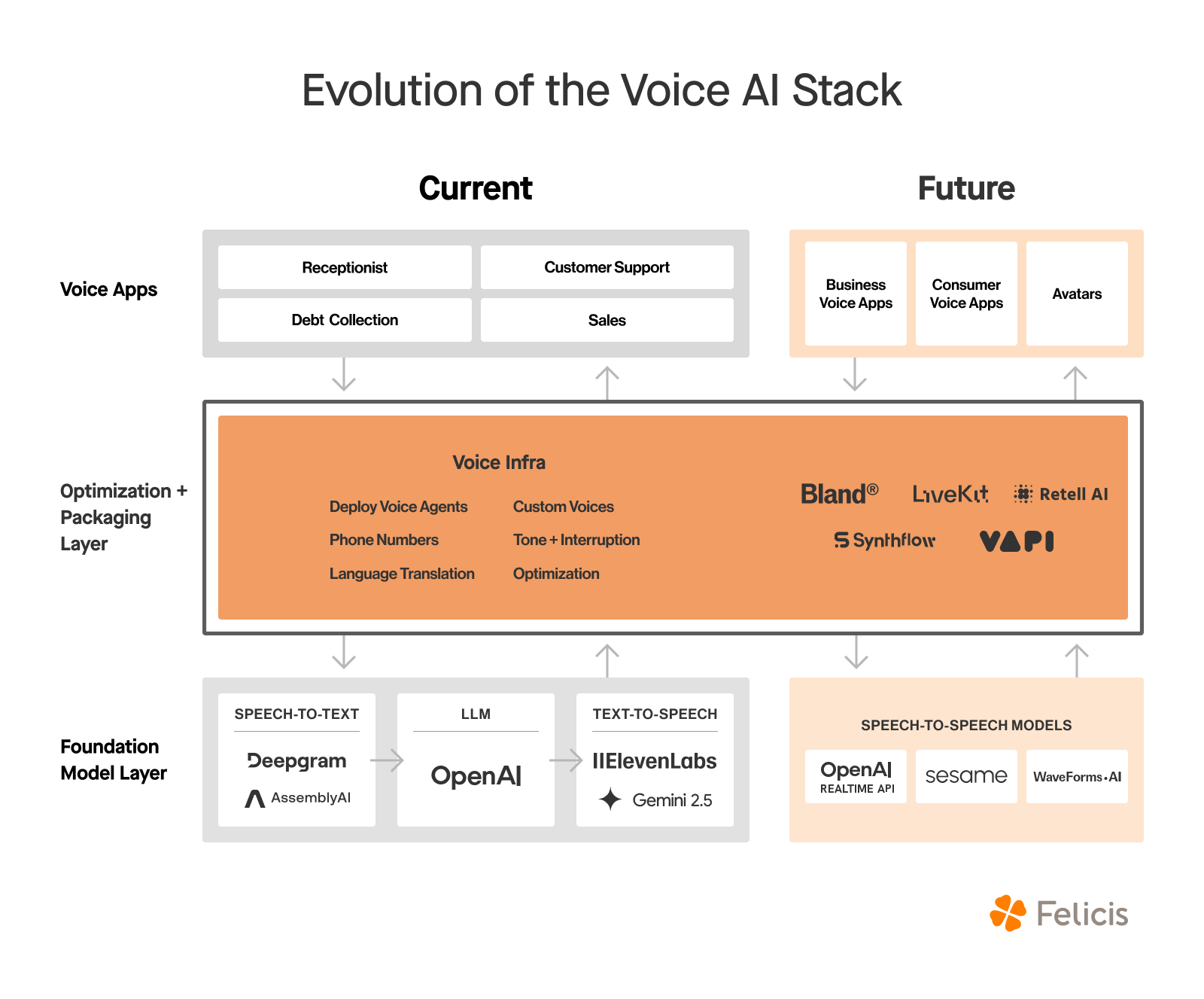
‍
There is also more to it than just packaging components. The real progress comes from improving latency and accuracy through fine-tuning, prompting, and feedback loops. These techniques are required to truly solve for areas where voice AI still struggles, such as:
- Differentiating between multiple speakers
- Appropriate tone and intonation
- Management of crosstalk and cutoffs
- Memory/context handling across calls
- Correcting for background noise
- Better understanding of unique business context
The massive voice AI opportunity exists in packaging and optimizing the voice stack into a streamlined, developer-friendly platform. Just as Twilio and Stripe turned complex communication and payments infrastructure into simple APIs, new startups like Vapi, Retell, and Bland are doing the same for voice AI. By packaging all of the necessary voice AI components into more developer-friendly APIs, they’re increasingly looking like a new “Twilio of voice AI.”
In the near term, we’re seeing insatiable demand for these developer kits to power high-growth AI apps that span customer support, healthcare, sales, accounts receivable, scheduling, debt collection, and more. The scope is massive. Longer-term, there is also a clear path laid by Twilio to follow: start with developer kits that abstract away communication complexity, and gradually move up the stack to offer more of the application layer.Â
Zooming out further, voice is one of the primary ways humans interact with each other, and will ultimately interact with AI. This spans both consumer and enterprise applications. Looking ahead, we also see real-time avatars as a natural evolution of the AI access layer. But again, low-latency, high-fidelity voice AI is also key to making avatars work.
The massive near-term opportunity to simplify voice complexity with developer kits, paired with the longer-term potential to move up or down the stack and power emerging technologies like avatars, signals that we’re at a true inflection point in voice AI. Taken together, it’s one of the most exciting and underexplored frontiers in AI infrastructure and is ripe for founders to build what's next.
‍
Thanks to Daniel Cahn of Slingshot for his feedback on a draft of this post.